CDex Opportunities: What They Are and Why They are so Valuable
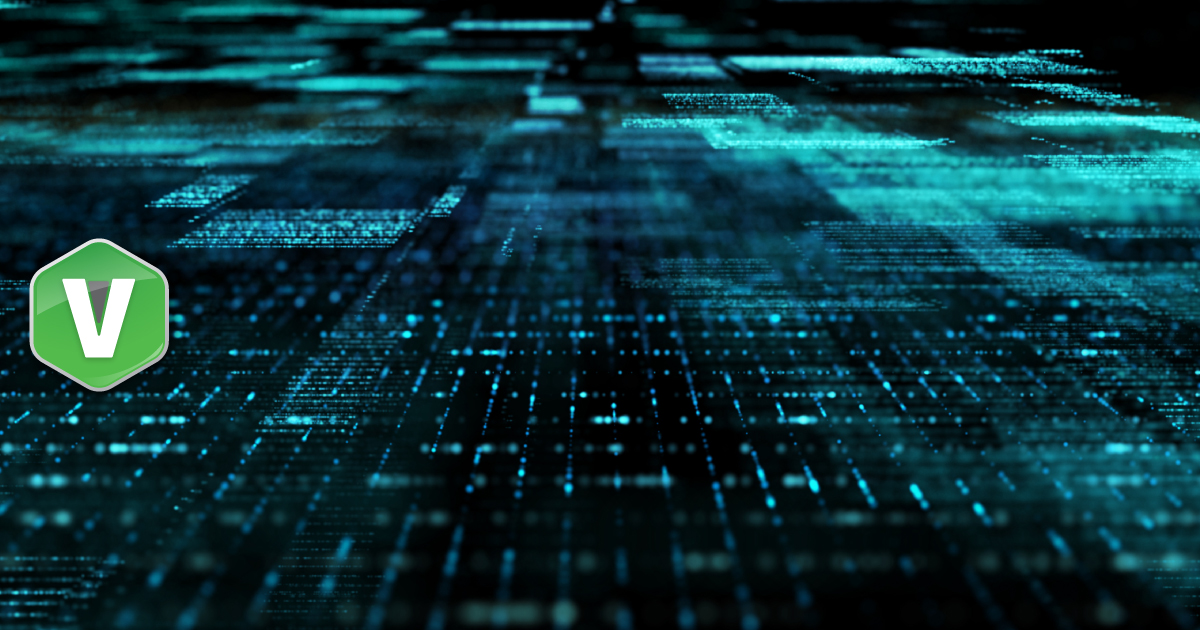
In a previous blog, we discussed the concept of data channels and how to score them on a number of meaningful parameters, giving us a standardized and benchmarked way of assessing Payers’ clinical data operations. Using data channels as building blocks and complexity scores as building blocks, we are able to objectively assess existing operations, both from an internal and external perspective.
In almost every payer operation assessed as of the time of this writing (approx. 80 health plans), complexity scores are high, meaning that there is significant need to improve. Complexity scores allow us to quantify two key aspects of existing data operations:
- Room for improvement of internal data operations: the makeup of the score allows us to point directly to the areas, channel-by-channel, and in the aggregate, where improvements can be made.
- The shortfall of the external portion of data channels vis-à-vis what is considered optimal. The higher the complexity score, the more desirable it is to replace that data channel, or at least improve on it, if possible.
Opportunities are defined as all the actions that can be taken to reduce complexity scores, internally and externally, plus one additional category:
- Internal Opportunities mirror internal complexities. With one minor exception, each factor that contributes to the internal complexity score, represents an opportunity improve and therefore also contributes to the opportunity score.
- External Opportunities (Replacement) are those where an alternative to an existing channel with a high complexity score exists.
- External Opportunities (New) indicate that there is a new potential data channel from a source where there is not an existing structured data exchange (channel) at all.
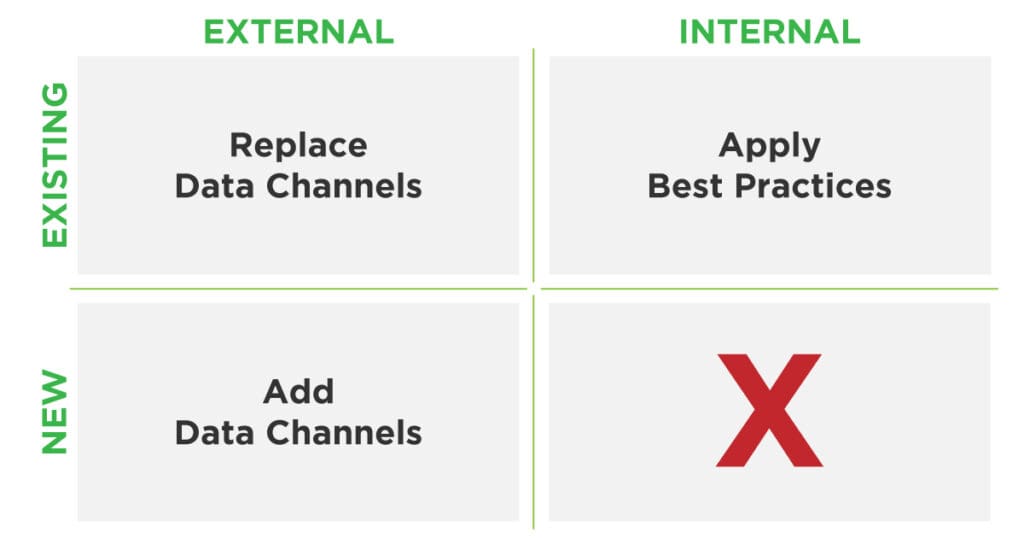
Figure 1 – Types of Opportunities
Opportunities, just like existing data channels, have scores: opportunity scores and weighted opportunity scores, which allows for a meaningful, standardized way to quantify the opportunities, one at a time and in the aggregate.
Scoring opportunities is a great step forward, especially since they existing in the context of a matching complexity scoring scheme. But that alone is not enough.
For opportunity scoring to be truly actionable, two more factors need to be considered: ROI and data completeness.
- ROI: acting on opportunities typically incurs costs. They can be IT investments, labor costs, data acquisition costs or a combination. Those costs need to be weighted against the benefits of implementing an opportunity, which include benefits that are specific to one or more use cases, operational savings and savings on data acquisition.
- Completeness: this metric attempts to determine what it means to have all relevant data for one or more use case and then attempting to attribute those data needs to opportunities. While there is no perfect way to predict what constitutes ‘complete’ and how to attain such completeness from available opportunities, approximation models are in development.
The combination of scoring complexity and having access to all the relevant opportunities, with the corresponding scoring elevates clinical data operations from a ‘best guess/best try’ effort to a model where all aspects are scored, benchmarked and the path to optimization is clear and measurable.